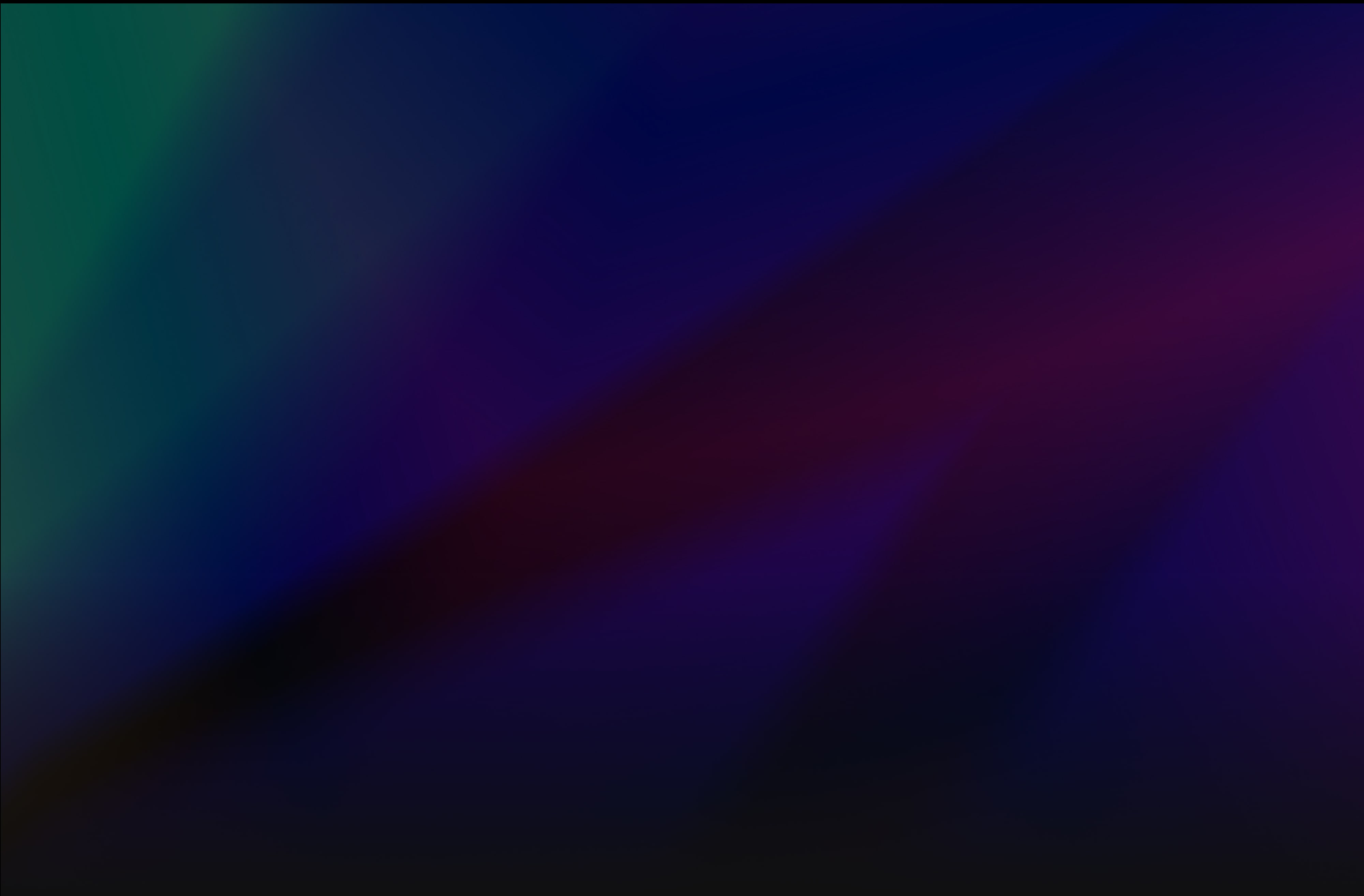
The best is yet to come
* This article is a continuation of our previous article that defined the concept and evolution of AI chips. In this article, we will explore key AI chip technologies and their market outlook. |
From learning to inference, From server to edge
In order to achieve the intelligence level of a smart human, we require new semiconductors for AI systems that can process a vast amount of data at a high speed, while still being power-efficient. If you want to have a glimpse of the upcoming technology that will shape our future, have a look at.
ㆍNPU(Neural Processing Unit): There is specialized hardware available that is designed to efficiently run artificial intelligence and deep learning algorithms. This hardware has a unique design for neural network computation, which is unlike CPUs and GPUs. It can handle complex mathematical operations, such as matrix operations and high-dimensional vector calculations, quickly and efficiently. ㆍNeuromorphic chip: It is a next-generation This is a new type of semiconductor that uses AI technology to simulate nerve cells (neurons) and connections (synapses) found in the human brain. Unlike traditional artificial neural networks, it operates using hardware to mimic neurons. This technology is commonly used for recognizing and processing unstructured data such as images and voice. ㆍFPGA(Field Programmable Gate Array): It is an intermediate form of programmable integrated circuit (IC), a type of non-memory semiconductor that can be reprogrammed for a specific purpose. It’s used to quickly process unique tasks that CPUs and GPUs can’t handle. ㆍASIC(Application Specific Integrated Circuit): It is a system semiconductor designed specifically to implement applications for specific systems. Unlike general-purpose processors, they are optimized for high efficiency and low power consumption. They are tailored to perform specific tasks such as Bitcoin mining or running particular algorithms. ㆍPIM(Processing In Memory): This technique involves performing some computations within the memory itself. When applied to AI computations, it can significantly reduce power consumption and increase data processing speed. This integration of memory and processor has the potential to revolutionize semiconductor design and change the way we approach computing in the future. |
Until now, AI semiconductors that come with AI accelerators have been primarily used to create AI servers. These AI servers have, in turn, been used to build AI data centers. However, in the future, high-performance AI semiconductors will not only run in data centers but will also be installed in our electronic devices such as smartphones, laptops, TVs, and refrigerators. This means that everyone will have a “small chip” in their lives.
AI is advancing at an accelerated pace, much like semiconductors. It has progressed from neural networks that analyze text using natural language processing techniques to multimodal, super-scale AI that can handle images, speech, and text simultaneously. Furthermore, we are on the verge of developing artificial general intelligence (AGI), which is capable of human-like reasoning and problem-solving abilities regardless of the topic at hand.
The AI semiconductor market is experiencing two significant changes. Soon, AI semiconductors will not only be utilized in data centers and high-performance servers but also in everyday devices like smartphones, cars, and home appliances. This will result in the emergence of “on-device AI,” which can function independently without an internet connection. Furthermore, as AI capabilities become more advanced, the demand for “inference” AI to service developed AI models is expected to skyrocket. The growth of the AI semiconductor market is moving from learning to inference and from the server to the edge.
Fast movers win the race
The market for AI semiconductors can be compared to an endless track, where the race has already begun. A runner’s life is all about speed. As in any race, speed is essential to stay ahead of competitors and overcome their weaknesses. According to Gartner, a global market research firm, the AI semiconductor market is predicted to grow to approximately $114.4 billion by 2027.
At present, Nvidia seems to be the leader in the AI semiconductor race. They hold a significant share of this market with their GPUs, accounting for about 90% of the GPUs in AI servers. Their distribution software, CUDA, has become a staple for developers designing AI chips. It is noteworthy that CUDA only runs on Nvidia’s GPUs, which has further strengthened their dominance.
It’s evident that Nvidia’s control over the AI semiconductor market is problematic. GPUs, which are designed for graphic processing, are their main offering. To address this issue, a new AI semiconductor ecosystem has emerged with the aim of disrupting Nvidia’s monopoly or creating better AI semiconductors. Several big tech companies, established semiconductor manufacturers, and startups in the semiconductor industry are at the starting line of the race.
Big tech companies like Google, Microsoft, and Meta have been creating their own AI chips to power their services. Intel has taken advantage of its dominance in the CPU market by launching a single chip that combines the benefits of CPUs and GPUs to enhance computing power. Meanwhile, Sam Oltman, the CEO of OpenAI (the company behind ChatGPT), is investing a massive $7 trillion to not only develop semiconductors but also to build a supply chain by expanding factories. Unlike other tech giants that only design AI semiconductors and outsource production to semiconductor manufacturers, OpenAI is likely to enter the production process itself.
Manufacturers of semiconductors are increasing their efforts to create cutting-edge AI semiconductors. This includes companies such as TSMC from Taiwan and Samsung Electronics and SK Hynix from South Korea, all of whom have their own semiconductor production facilities. TSMC and SK Hynix, the two largest foundries in the world, have formed an alliance to develop next-generation HBMs. Samsung Electronics, which claims to be the only integrated device manufacturer (IDM) with a full range of processes from production, foundry, and packaging, recently received a $6.4 billion semiconductor subsidy from the United States. The subsidy will enable Samsung to build a facility in the U.S. for the latest advanced semiconductor manufacturing technology, the 2nm (nanometer) process, and begin mass production next year.
Startups, not big tech companies or chipmakers, may be the game changers in the AI ecosystem. They move faster and exploit weaknesses in the existing market. They are particularly interested in developing chips that are cheaper and consume less power than Nvidia’s GPUs, which are expensive and power-hungry. With AI being actively developed in multiple sectors such as healthcare, finance, education, food, transportation and logistics, there is a growing need for chips that are tailored to specific purposes. By developing such chips, startups can reduce power consumption as fewer parameters mean less data for the LLM to calculate, resulting in lower carbon dioxide emissions. Startups that can successfully tackle the power consumption problem will have a major advantage in the on-device AI market, especially as the market shifts towards edge computing. (GPUs are less effective for edge computing as they tend to overheat.)
It is certain that to take the lead in the AI semiconductor market, companies that move fast will have an advantage. These companies are called “fast movers”, and they are not necessarily the first in the market or the second, but those who capture the market the fastest without being the first. (While a first mover is usually the leader, and a fast follower is the second in line.) Many semiconductor startups are examples of fast movers. How will they set new records in the AI semiconductor race, develop innovative solutions, and create decisive momentum?
The race is on. The time for startups is coming soon. Drum roll, please.