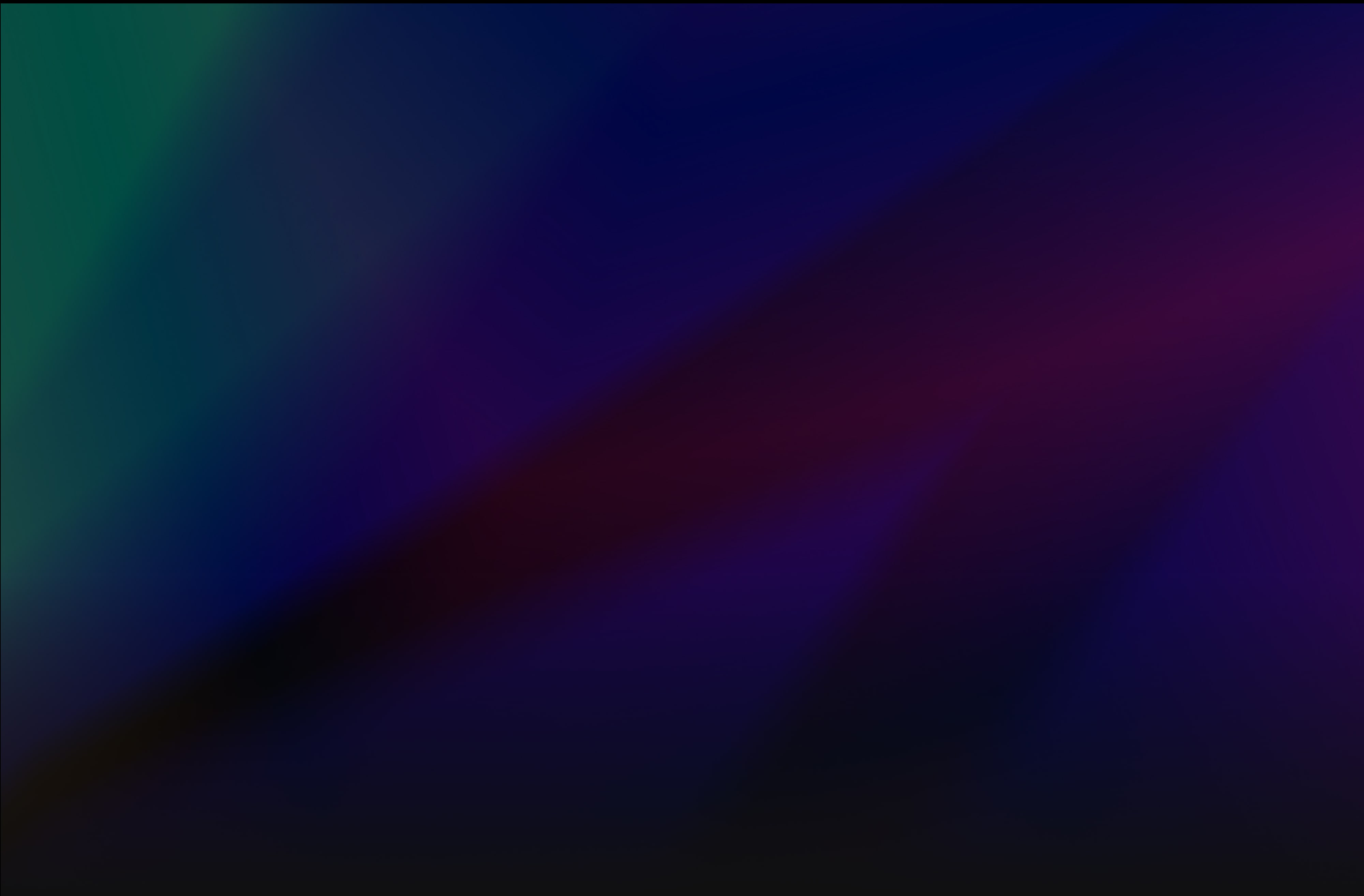
Why are small chips all the rage?
Artificial intelligence technology is part of our daily lives today. Facial recognition, controlling your smartphone with your voice, real-time translation, and more are all possible thanks to AI. If you’ve ever wondered how AI is able to make small talk with humans, create videos and music from a few sentences, and even detect and treat diseases in patients, you might be wondering who’s in charge of its brain. If you want to know the secret, look inside the AI’s brain. What do you see? A tiny chip.
It’s embedded with ‘AI semiconductors’
When asked “What animal do you see there?”, the difference in the thought process between humans and AI becomes apparent. While a human might examine a cat’s whiskers, pointy ears, and overall appearance to determine that it’s a cat, AI might analyze data from learning about many animal faces and conclude that it’s most likely a cat. In order for AI to think and communicate like humans, it must process a large amount of data and make its own inferences. To do this, special processors known as “AI semiconductors” are required, which can perform large-scale computations at ultra-high speed and ultra-low power. (By ultra-power, we mean focusing on minimizing power consumption to extend battery life and increase energy efficiency.) In other words, AI semiconductors must be integrated into the brains of AI to realize the AI services we enjoy today.
Semiconductors are a type of material that possesses unique properties that allow it to act as both a conductor and a non-conductor of electricity, depending on specific conditions. This characteristic makes it possible to use semiconductors in electronic devices. There are two primary categories of semiconductors: memory semiconductors that store information and non-memory semiconductors that are primarily used for information processing operations. Memory semiconductors include RAM, ROM, D-RAM, and NAND flash, while non-memory semiconductors include CPUs and GPUs. When it comes to AI semiconductors, we can define non-memory semiconductors as computation-oriented semiconductors that process data and algorithms necessary for implementing AI services. Non-memory semiconductors are used in a similar fashion to system semiconductors.
AI semiconductors consist of three main components: D-RAM, which stores information, HBM, which is created by stacking D-RAM, and GPU. These semiconductors are also referred to as “AI accelerators,” as they are specifically designed to efficiently process AI computations. AI accelerators can handle large amounts of data and perform complex calculations to speed up the learning and inference of AI models.
How it’s evolving
Scientists have been attempting to replicate human neural networks for several decades now, leading to the development of the Artificial Neural Network (ANN), which is designed after the human brain. The ANN comprises nodes and layers that structure deep learning AI. Within this multi-layered structure, mathematical operations allow over tens of billions of parameters, which are the various variables that AI considers for computation, to interact. For instance, ChatGPT’s third-generation model possesses 175 billion parameters, whereas the number of parameters for the fourth-generation model remains undisclosed, though it is estimated to be as high as 100 trillion, which is equal to the number of synapses in the human brain.
GPUs are chips that specialize in processing large amounts of graphics information in pixels. They are known for their ability to process data in parallel, which makes them ideal for handling the simultaneous operations of neural networks. In the past, CPUs, or central processing units, were responsible for input, output, and instruction processing in computers, but they processed data in series, which made it difficult to work with neural networks. GPUs were originally developed for graphics computations with large amounts of data, but they became the savior of AI due to their ability to process data in parallel. However, they have their limitations because they were not designed specifically for AI computation. That’s why intelligent semiconductors, which are optimized for AI algorithms, are emerging as a new type of AI-specific semiconductor.
Neural networks demand a large amount of storage as compared to traditional software. GPUs are computational devices, which means that they require a separate device to store the neural networks. This becomes especially significant in edge systems since the neural network and the storage device for the training data need to be kept in close proximity. Memory semiconductor companies are now developing memory chips, such as HBM, which can provide high-capacity, high-bandwidth memory.
AI and semiconductors are closely intertwined, just as the brain and intelligence develop together. Advancements in semiconductor technology are crucial for improving AI performance, and the global market for AI semiconductors is rapidly growing.
“Who will evolve faster?”
* In this article, we define the concept and evolution of AI chips and discuss key AI chip technologies and the market outlook in the next installment. |